What thing can you take back to your business to implement that will help you? There isn’t one and that is the point. They were successful because they implemented them all. All.
Since human beings began growing crops in a field to establish communities, the mantra has always been “grow more”. Growing more means more food, more stability, and (of course) more money. That money means better equipment, more land, and a means to retire and let the next generation take over. However, one company is showing farmers how to increase profit margins through the use of data.

However, with the advent of technology the time honored tradition of growing more took an odd turn. Corn is an industry that measures success in bushels per acre. In chasing more bushels per acre more inputs are needed to push crops to new levels. Farmers may even go bankrupt trying to get a 20% increase in bushels just to better their neighbor or hit a projection.
The advent of technology has changed the paradigm in how crops are cultivated and how they can be grown. Keep in mind, people were still using livestock to plow fields as late as the 1950’s. Now they use ultra precise GPS to navigate a family farm they know like the back of their hands, all for more bushels per acre at harvest time.
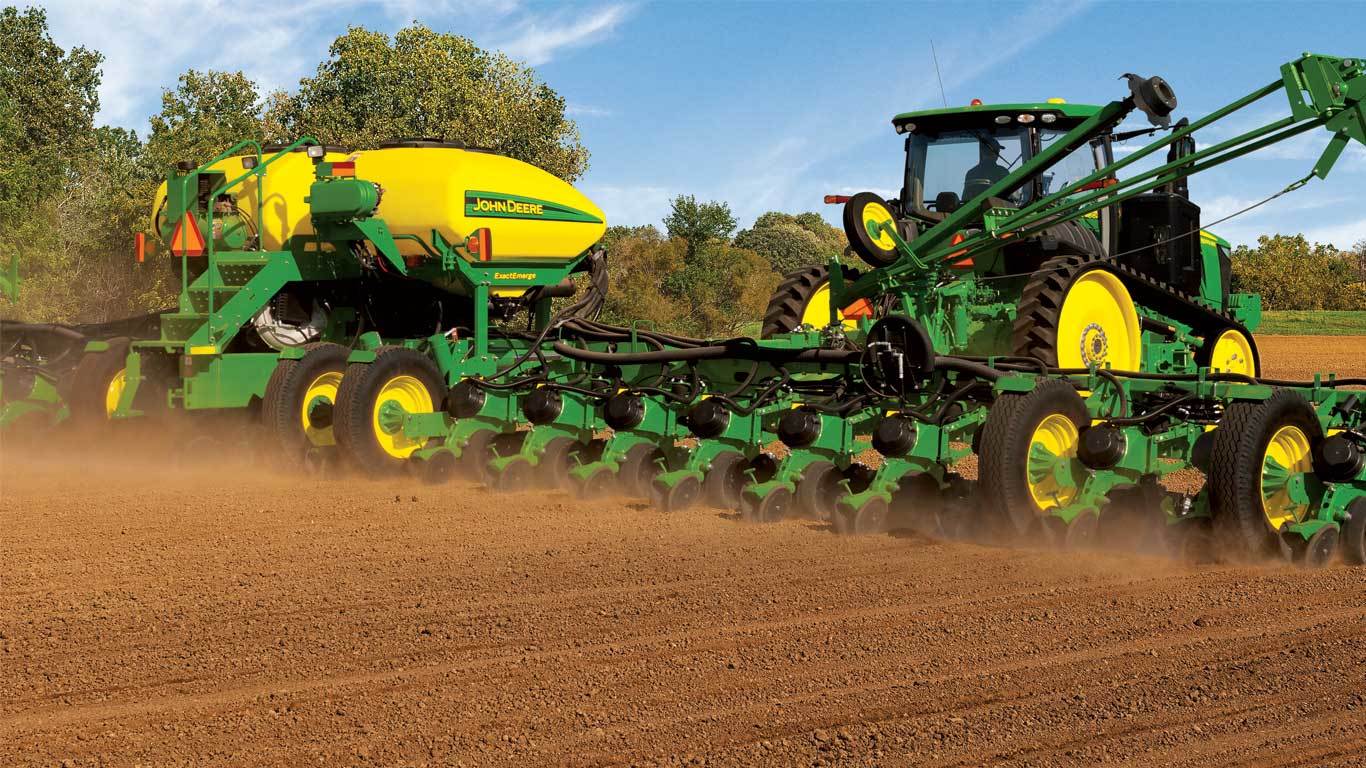
With the quest for more bushels comes the additional costs and “inputs”. Inputs can be anything you put into a field to help it grow: water, fertilizer, herbicide, pesticide, and more are all inputs. With deep enough pockets you can fund any number of thousands of variables that go into a particular field. But are those inputs really helping you make more money?
Working Smarter not Harder
One company, Crop Tech Solutions, realized you can work smarter and not harder. As it turns out being too productive and chasing higher bushel counts can actually be detrimental to your bottom line. Its such a foreign idea let me write that again another way. You can make more money, from less bushels per acre.
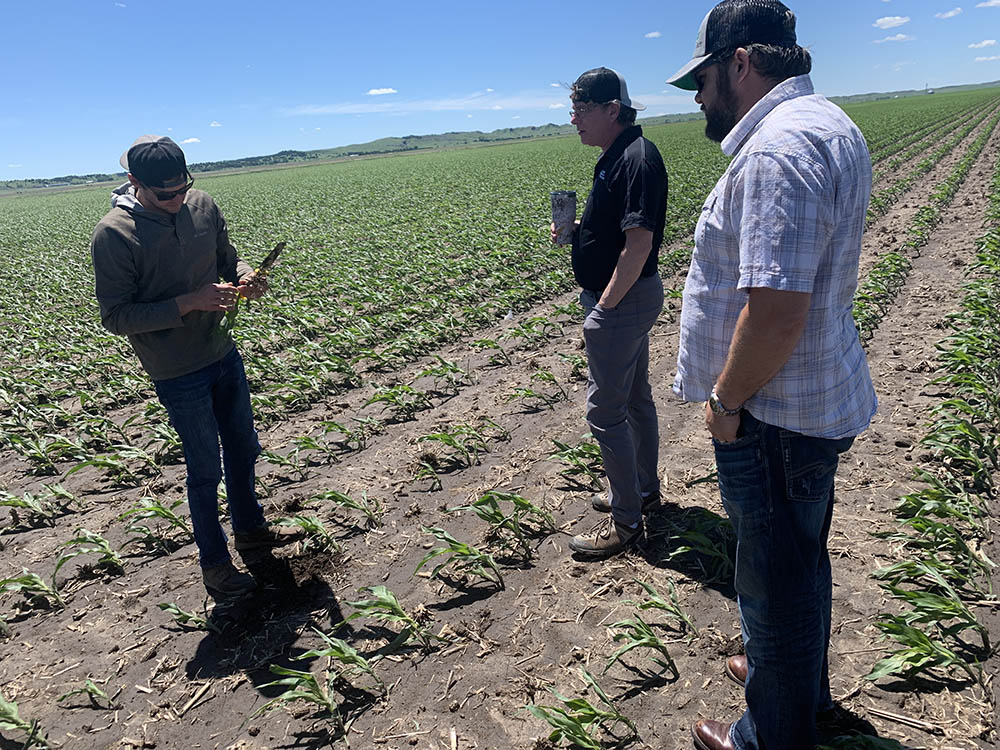
Seed companies want to sell more seeds, so more seeds means more profit…but for whom? The soil and field conditions will dictate how the corn grows in the field, not the seed count going in. The more you have to add to that field in terms of inputs the more money it will cost to grow it. Each input erodes your profit margin and if its a down year for corn pricing, you’re in the red pretty fast.
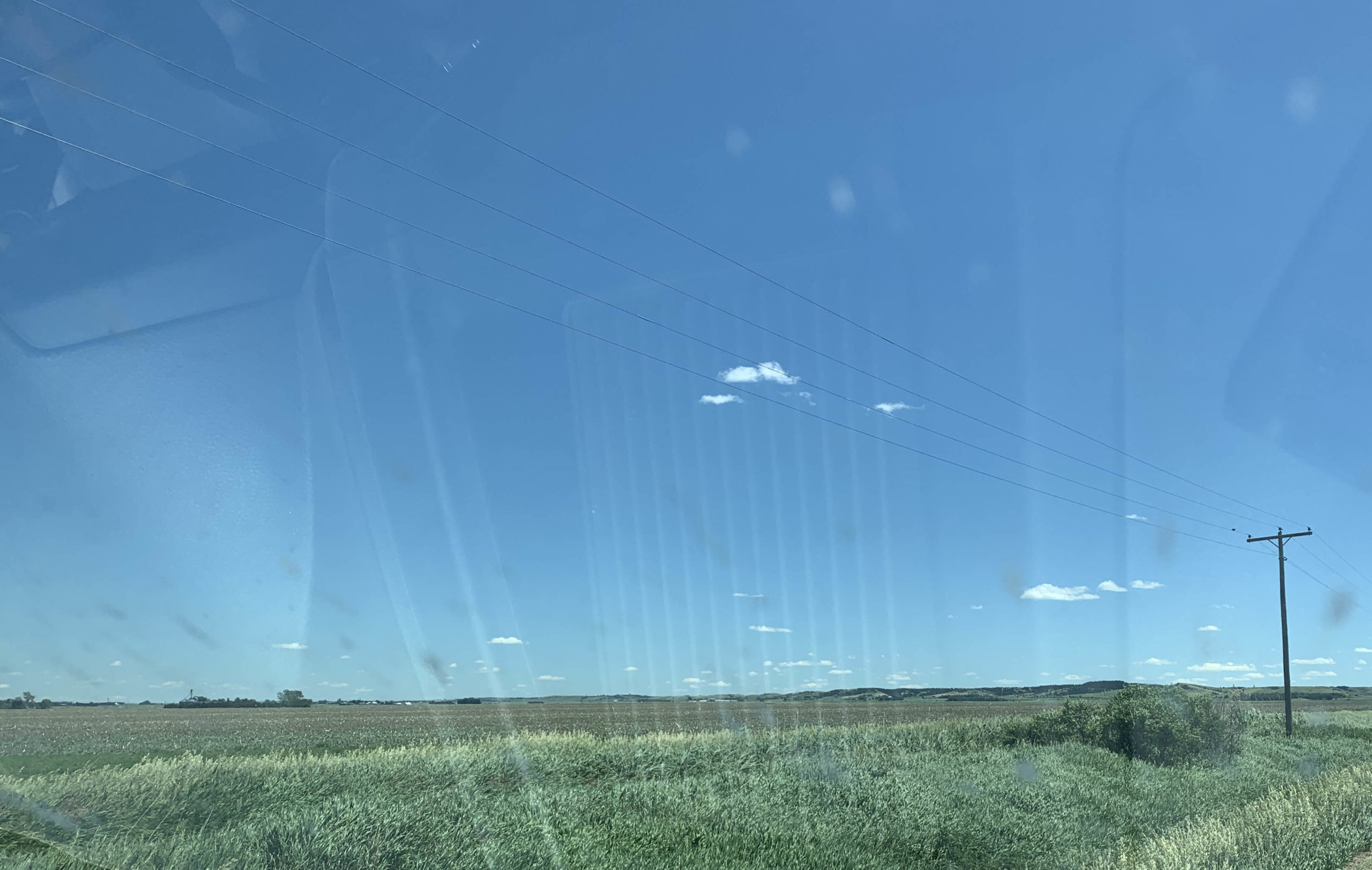
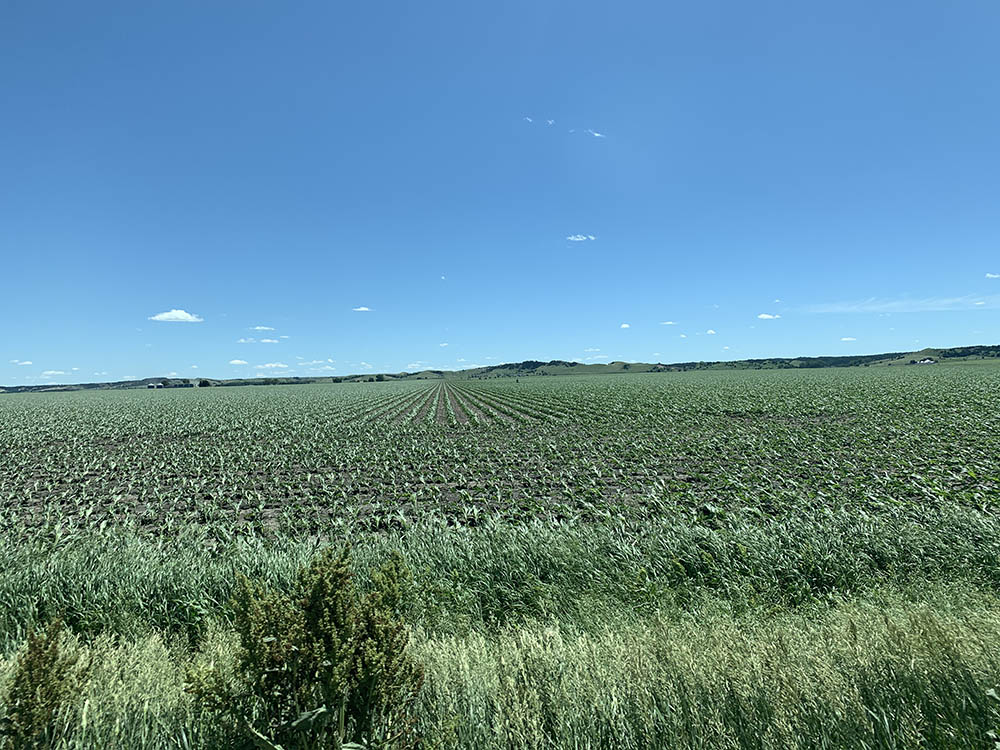
So, somewhat counter intuitively, you can make more money farming with less bushels per acre. By using the data and the technology for precision planting you maximize the potential of the plants without competing for resources. Farming smarter, not harder, the plants can reach a greater potential without competing for resources.
Growing with CAN
Contemporary Analysis has been working with Crop Tech Solutions to get even more from their data. The more effective their data collection and prediction, the easier it is for their customers to see significant gains in profitability. Crop Tech Solutions has been able to help local farmers find Millions in efficiencies, and shows no signs of stopping any time soon.
Crop Tech delivers customized prescriptions for each field. Based on the data points from various aspects of farming. As they layer additional data to the process, the more they are able to predict the best combination of field characteristics and inputs. Their prescription helps crops grow to their fullest potential without wasting input dollars trying to push it along.
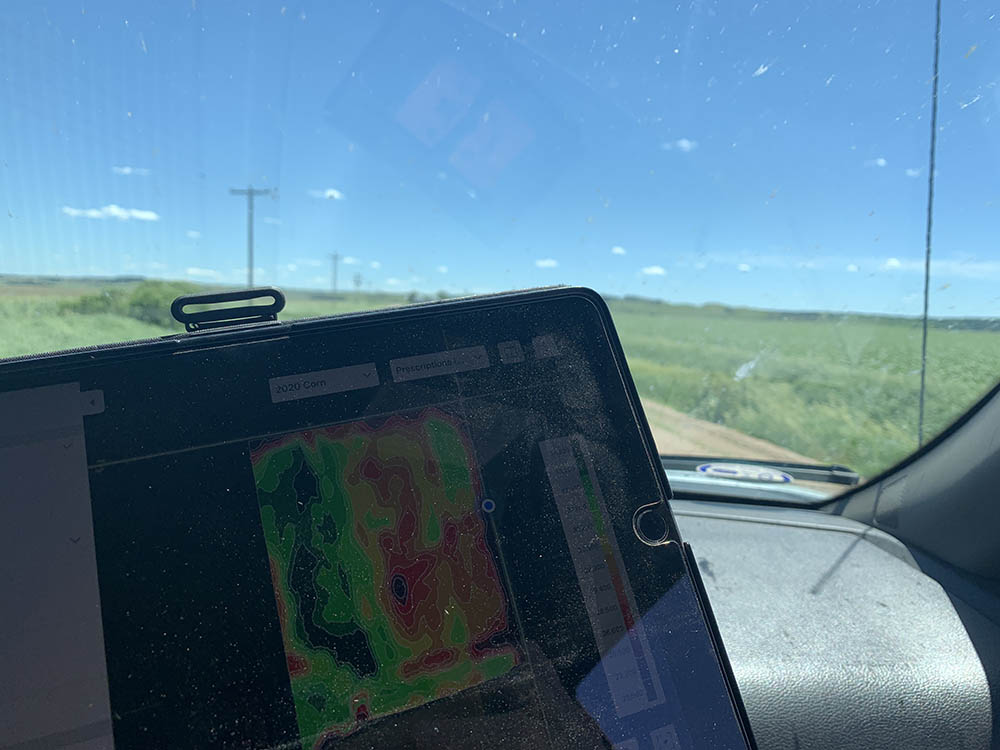
So how do you get more profit from LESS bushels per acre? It all comes down to how much you have to spend to grow the corn. To get 250 bushels per acre you have to purchase chemicals, fertilizer, fuel, and more. These inputs are often in significant quantities to “push” the field to that bushel target. Plants have to compete for resources so they are strained and don’t grow to full potential. Resources like water, sunlight, and root size can dramatically change the way your crops grow.
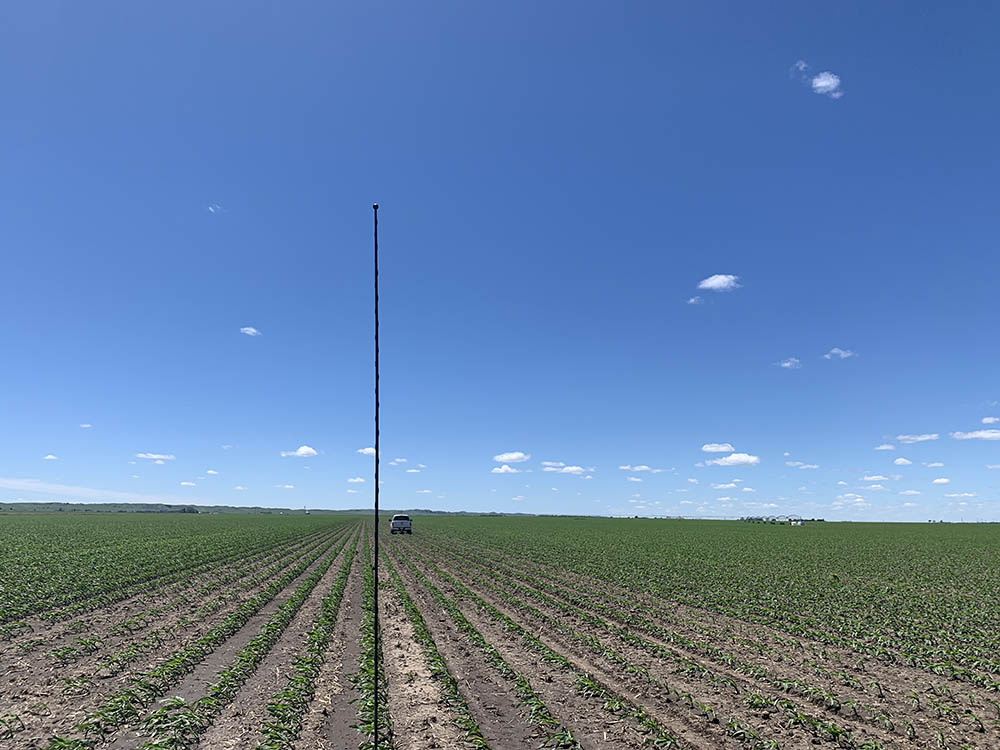
When you use the data to understand what the ground can carry, down to the inch in the field, you can optimize every row to get monster corn that isn’t struggling for limited resources. When the corn can grow to its full potential, you don’t have to spend thousands of dollars trying to push it along. The data takes the “gut feeling” out of farming.
Precision agriculture delivers results, but only if you know how to deploy it. You can have the best equipment in the world, but if you don’t set it up right you’re wasting money. The prescription delivered from the layered data points helps program seed planting equipment (for example) accurately. A section of your field may call for a 6 inch gap between plants while another segment, 20 feet away, may call for 1 1/2 inches between plants. The compulsion to “split the difference” and then “set it and forget it” could cost you hundreds of thousands of dollars in the long run. You’re literally betting the farm on how many inches apart you place crops in the field.
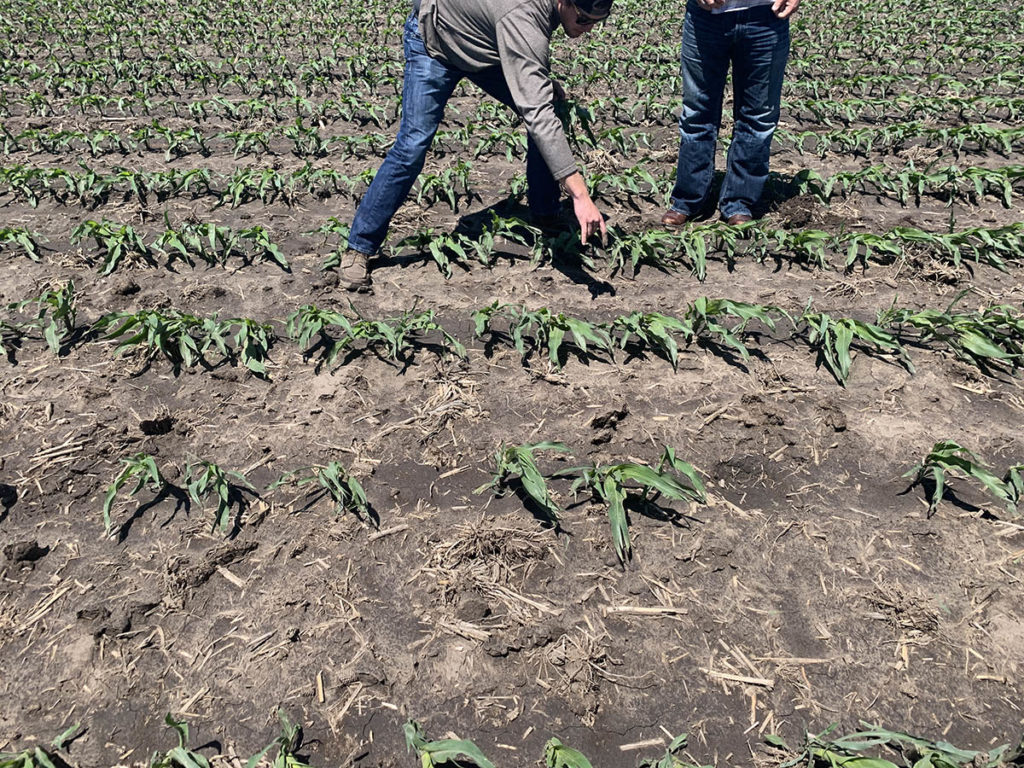
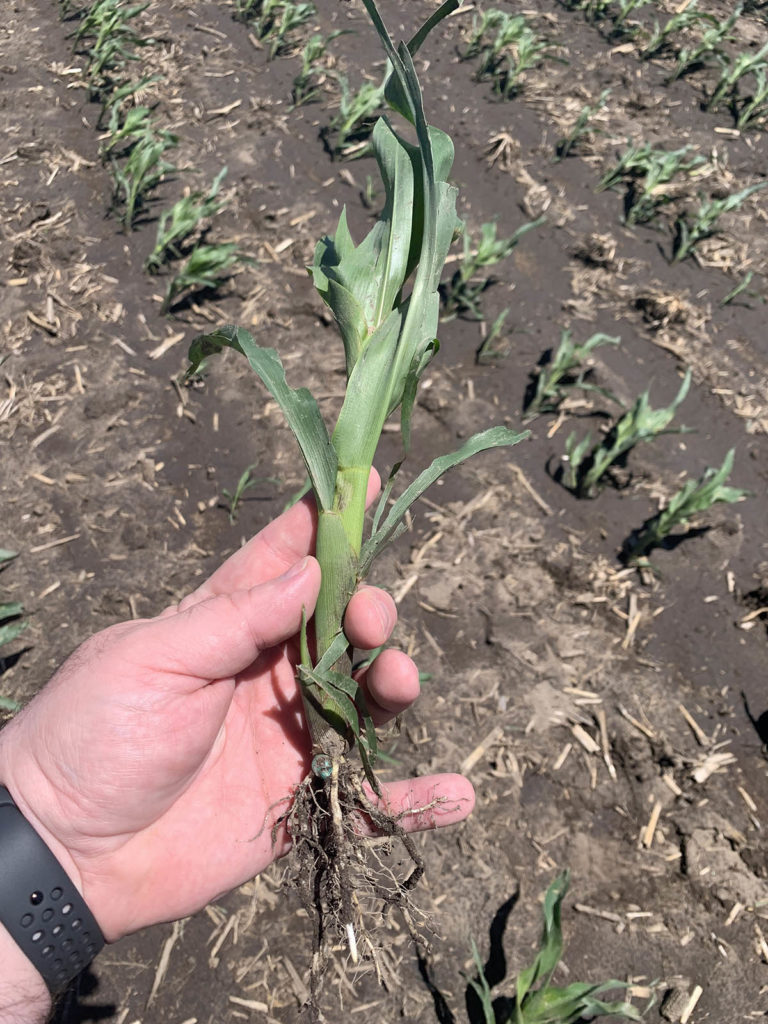
Very few farmers work on an all cash basis. Each year they take a loan from the bank to fund the operation. These loans can be in the hundreds of thousands or even millions of dollars. The less you can spend on inputs the less you need to borrow. The less you need to borrow, the less you have in interest payments. When you go to sell your crops the fluctuating prices mean the farmer who grew quality crops with lean inputs will see more profit. The farmer who grew to the seed company suggestions has a skinnier margin (if any) and could actually lose money growing corn in a bad year.
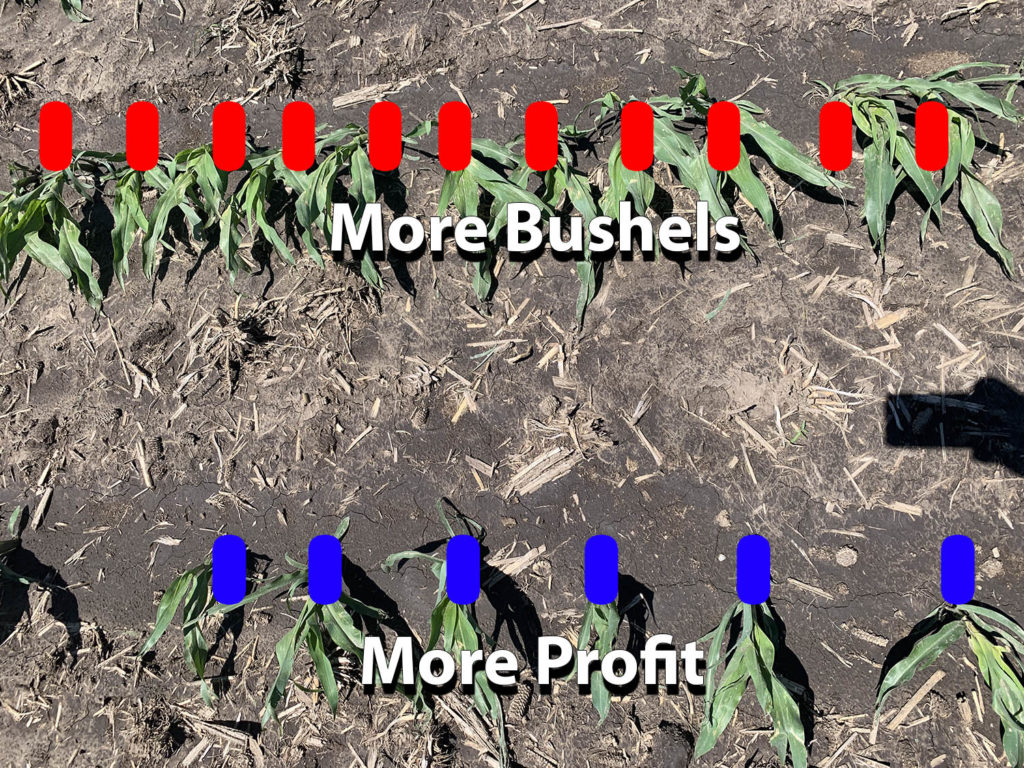
The Payoff
At Contemporary Analysis we love working in what we call the “Steel toe industries”. These areas include, Agriculture, Construction, Manufacturing, Transportation, and Logistics. We carry over lessons learned from our non-steel toe clients and vice versa. The end result is a broad spectrum of options where others may only see one or two.
Our goal is to help companies make better data driven decisions at every turn. In this instance, its helping farmers remain profitable year over year. Because of the champions of data like Crop Tech Solutions we can help keep families and even entire communities thriving through data.
Overall we had a great visit with the guys from Crop Tech Solutions. They even gave us a private tour of the Sod House Museum which is one of their passion projects.
If you’d like to see how we could help you do more with less, contact us.