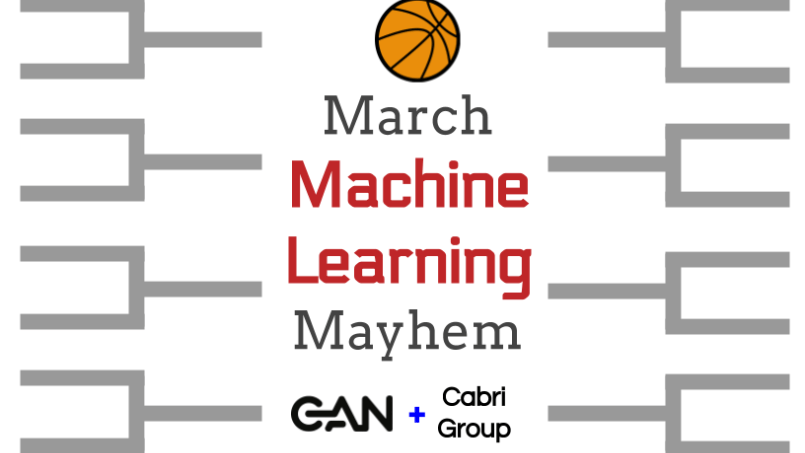
2018 NCAA bracket picks using Machine Learning.
Contemporary Analysis (CAN) and Cabri Group and have teamed up again to use Machine Learning to predict the 2018 NCAA Men’s Basketball Tournament. This is different than last year as we are picking the entire 2018 bracket instead of just upsets.
Historically, only 26% of tournament’s games end in an upset (this includes games from all rounds). That’s 17 out of 64 games. Last year we did really good. Only failing to predict 3 upsets and getting 50% of our predictions right. We are going to need to improve a bunch to win that 1M/year for life from Berkshire Hathaway–including that wee bit about having to work for Berkshire Hathaway to be eligible. This year we added far more variables and used an ensemble model. Will we be perfect? Probably not. Here is the problem with using Machine Learning to try and predict a perfect bracket:
A). Error propagates itself through the bracket. This is why the odds of a perfect bracket are around 1:128 billion. If you pick San Diego State to upset Houston-
Side note: The machine learning is in fact, picking Houston by the slimmest of margins. However, if San Diego State wins, the machine learning is actually picking them to go on to beat Michigan, Providence, and then Ohio State to win the entire region.
and then Houston actually wins, you will lose the entire region. Perfection may have to do with a 6/11 game that no one would normally care about except its the tournament, and everyone cares about every game.
B). Machine Learning and Predictive Analytics aren’t about being 100% accurate. You wouldn’t want to pay for that kind of accuracy even if it were possible. We are trying to be less wrong for companies. This is why predicting upsets made sense and the whole 2018 NCAA Bracket is so hard. Figuring out who is most likely to be an outlier (churn) is something we do all the time. And, we can error on the side of being wrong. We would just tell you to call both Houston and San Diego State (in this instance) because calling them to talk to them about staying at your company has no ill effect. (i.e. there is very little cost to being wrong in this example.) There is a huge cost to being wrong in the tournament in the later rounds as you are predicting the next game based on your assumption of correctly predicting the last game.
Without further ado, here is what the Machine Learning algorithm predicted as the bracket:
CAN Bracket-pdf
If you have questions on this type of analysis or machine learning in general, (or if we are perfect and you would like to congratulate us), please don’t hesitate to contact:
Gordon Summers of Cabri Group (Gordon.Summers@CabriGroup.com), or
Nate Watson at CAN (nate@canworksmart.com).
Now for some disclaimers:
Understand the technique that finds a group of winners (or losers) in 2018 NCAA bracket can be based on any metric. Our analysis isn’t to support gambling, but to open up people’s minds onto the possibilities of leveraging Machine Learning for their businesses. If we can predict things as seemingly complex as a basketball tournament (Something that has never been correctly predicted), then imagine what we could do with your data that drives your decisions
We will be keeping score using the very traditional 1,2,4,8,16 point process.
**Any handicapping sports odds information contained herein is for entertainment purposes only. Neither CAN nor Cabri Group condone using this information to contravene any law or statute; it’s up to you to determine whether gambling is legal in your jurisdiction. This information is not associated with nor is it endorsed by any professional or collegiate league, association or team. Machine Learning can be done by anyone, but is done best with professional guidance.