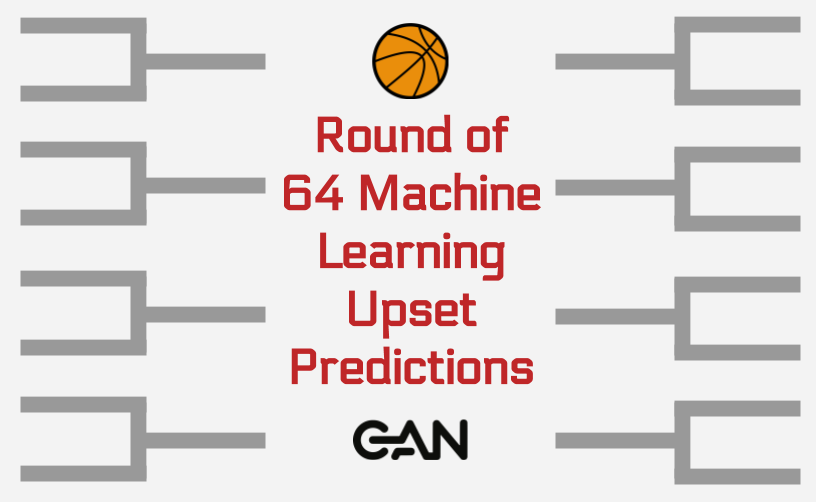
2017 NCAA Tournament Round of 64 Upset Predictions
The Cabri Group / CAN Machine Learning Lower Seed Win Prediction tool has made its first round forecast! Without further ado:
East Tennessee St. (13) over Florida (4)
Xavier (11) over Maryland (6)
Vermont (13) over Purdue (4)
Florida Gulf Coast (14) over Florida St. (3)
Nevada (12) over Iowa St. (5)
Rhode Island (11) over Creighton (6)
Wichita St. (10) over Dayton (7)
* If the last play in games add another predicted upset, we’ll update that prior to the game starting.
Update: USC (11) over SMU (6)
One of the obvious observations on the predictions is: “Wait, no 8/9 upsets????” Remember these games show the most similar characteristics of the largest historic collection of upsets. This doesn’t mean that there will be no upsets as 8/9 nor that all of the predictions above will hit (remember we are going for 47% upsets) nor that all games not listed will have the favorites win. The games on the list are there because they share the most characteristics with historic times when the lower seed won.
Also, one of the key team members on this project, Matt, is a big Creighton fan (and grad). He was not happy to see Creighton on the list. I’ll speak to that one specifically. In the technical notes, I indicated that one of the many criteria that is being used is was Defensive Efficiency (DE). Machine Learning algorithm (Evolutionary Analysis) doesn’t like it when the lower seed has a large gap of DE between the lower seed and the higher seed. Creighton actually has a lower Defensive Efficiency than Rhode Island. Sorry Matt. Again, it doesn’t mean Creighton won’t win, it only means that the Rhode Island v. Creighton game shares more criteria with a the largest collection of historic upsets than the other games in the tournament.
As we indicated, we will use the odds as well as a count of upsets to determine how well we do as the tournament goes on. We’ll have a new set of predictions on Saturday for the next round of the tournament and a recap coming on Monday.
For more information about how we created the Machine Learning algorithm and how we are keeping score, you may read the Machine Learning article here:
http://can2013.wpengine.com/machine-learning-basketball-methodology