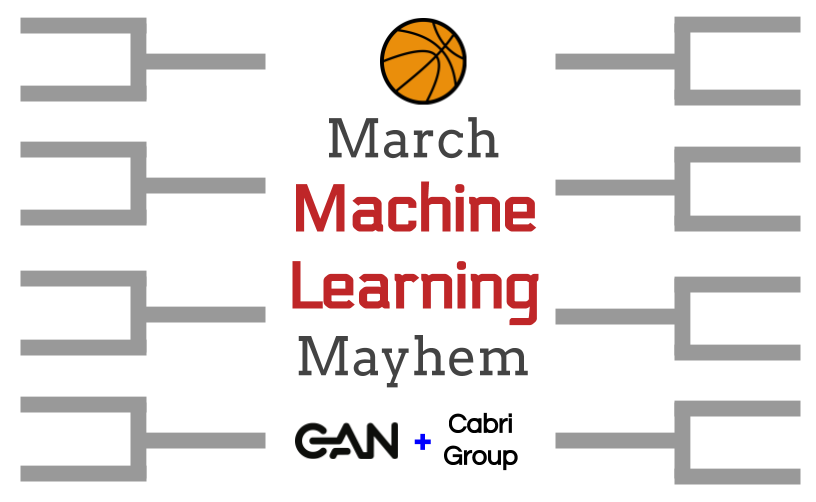
Machine Learning Upset Prediction Project Proves its Value
At the beginning of the project, we set out to show how the 2017 NCAA College Basketball Tournament could be a proving ground for Machine Learning analysis. There are very few places in the world where we can use the same model to predict multiple outcomes in a short period of time, have a ready-made scorecard (Vegas), have the general public understand what we are trying to do, and have a chance to “beat” the algorithm with their own knowledge.
You could say our findings have been a “Slam Dunk” (I couldn’t help myself).
Before diving into the results, I wanted the reader to understand what we were up against. It’s easy to pick chalk (always picking the better seed). In fact, that is how the games are supposed to work. The 8 seed is supposed to beat the 9. And for the most part, the NCAA does a decent job. Historically, only 26% of tournament’s games end in an upset (this includes games from all rounds). That’s 17 out of 64 games. This was never going to be easy.
Project Recap
We predicted 20 upsets and got 10 right (50%). We only missed predicting 3 upsets.
Using Vegas as a scorecard and having bet $100 “dollars” on each predicted upset, we would have ended up +$2,605 off our simulated bets (a 30% ROI)–the majority of this coming from long shot underdogs.
Think about this. If we would have bet all chalk on games except the ones the algorithm predicted as upsets, then out of 61 games we would have only missed 13. That’s 79% accurate!
Let’s look at this another way. Our algorithm predicted 77% (10/13) of something that is only 26% likely to happen in the first place. Now think about what you would do if you could identify an unlikely event in your business with 77% accuracy.
- What would you do if you knew 77% of the customers who were going to leave before they left?
- What would you do if you knew 77% of failed batches before they happened?
- What would you do if you knew 77% of your plant’s machine failures before they happened?
Business Scenario
You have a theory that some of your clients would buy more “product” if they were called and offered an upgraded deal. However you don’t want to call all of your clients because you have so many. What you do have is a dataset of past customers that successfully responded to this type of nudge. Using your data, our machine learning algorithm could predict a set of your clients that would be 77% likely to purchase more product if called.
Game changer right?
Why this is huge
Our Machine Learning lower seed winning project was looking to predict as accurately as we could a lower seeded team winning in the NCAA tournament. Our stated goal from the beginning was to get 47% of our picks correct and a mere 10% ROI. We beat both of those goals. Our Machine Learning algorithm, which uses a custom optimization engine called Evolutionary Analysis, looked at a comparison of 207 different metrics of college basketball teams and their results in prior tournaments. It selected ranges of those 207 measures that best matched up with historic wins by lower seeded teams. We then confirmed that the range was predictive by testing the selected ranges against a “clean” historic data set. This comparison is how we got our goal percent and ROI. We then published our forecasts before each round was played – the results speak for themselves.
While we still have 3 games to go, our initial point that Machine Learning can help you be better at making decisions from your data has been proven. Implementing Machine Learning isn’t hard so long as your business has these three characteristics:
- A data set with a large number of characteristics
- A measure of success to optimize upon
- A desire to learn from data to make changes in your organization
If this sounds like something that your business could use, please contact Nate Watson of CAN (Nate@CanWorkSmart.com) or Gordon Summers of Cabri Group (Gordon.Summers@CabriGroup.com) today.
Prediction Results
Here is a summary of our picks from the beginning of the project ($ indicates our successful pick where “money” was made):
East Tennessee St. over Florida
$ Xavier over Maryland
Vermont over Purdue
Florida Gulf Coast over Florida St.
Nevada over Iowa St.
$ Rhode Island over Creighton
$ Wichita St. over Dayton
$ USC over SMU
$ Wisconsin over Villanova
$ Xavier over Florida St.
Rhode Island over Oregon (tied with a minute to go)
Middle Tennessee over Butler
Wichita St. over Kentucky (tied with a minute to go)
Wisconsin over Florida (OT last second shot)
$ South Carolina over Baylor
$ Xavier over Arizona
Purdue over Kansas
Butler over North Carolina
$ South Carolina over Florida
$ Oregon over Kansas
And for those who are curious, our algorithm has detected one Final Four upset for this weekend:
Oregon over North Carolina
For more information about how we created the Machine Learning algorithm and how we kept score, please read our Machine Learning technical document. Additionally you can find results for the whole tournament here.